I had this bizarre dream last night: a row of old vending machines, each dispensing not snacks but people—tiny figures scuttling out as if answering a summons they never quite understood. Some machines had rusted levers and squeaky spouts, others sported blinking neon panels and digital keypads.
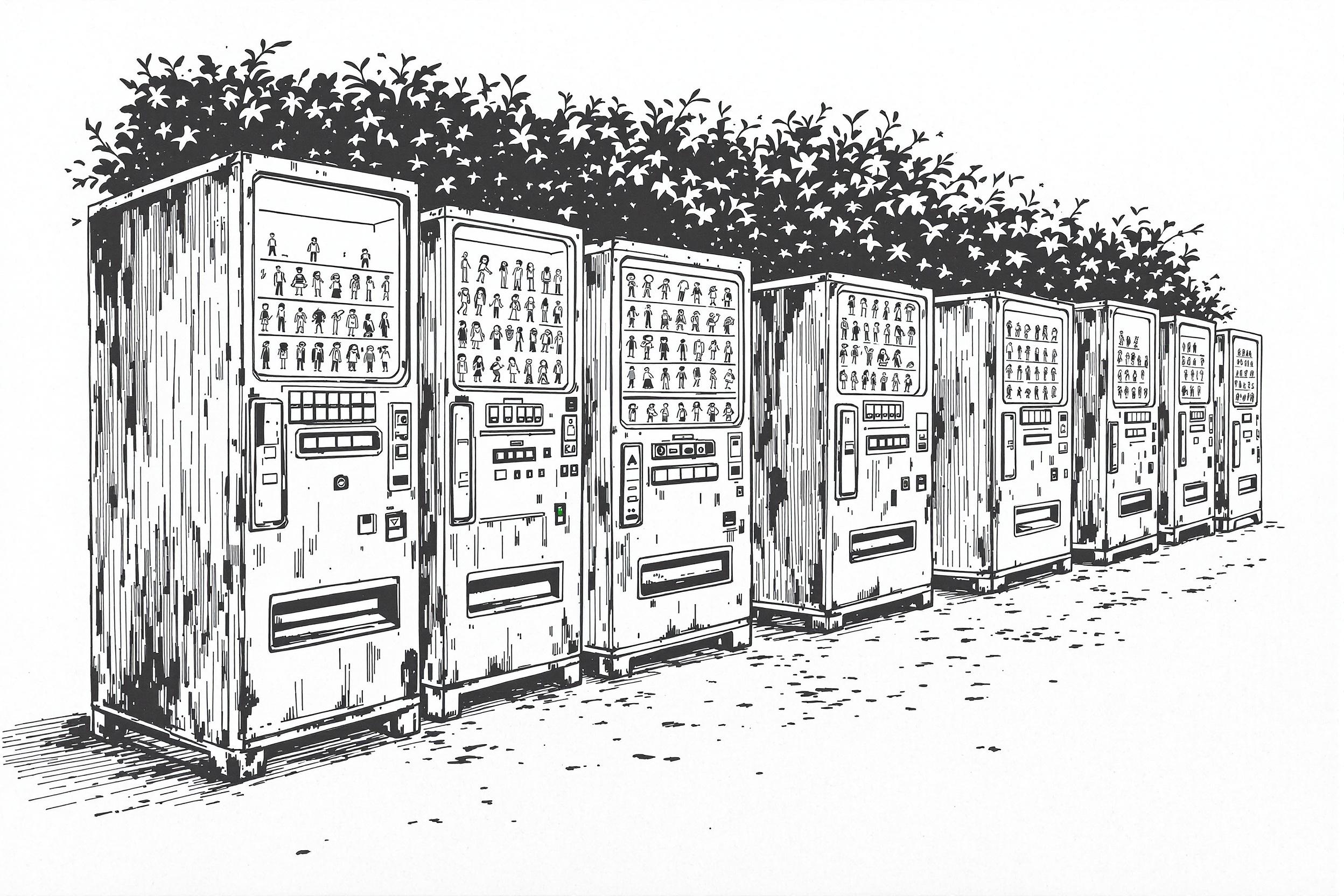
In the dream, I had a handful of tokens and tried to guess which machine would dispense the most suitable “person” to fill a mysterious role. Every time I guessed wrong, someone sprinted off into the night shouting, “You never knew me!”
It was absurd, but upon waking, it made me think: if only I’d had a way to predict which vending machine would release the right person before I spent a token. Oddly enough, this image reminds me of predictive analytics in modern recruitment—except, of course, we’re not dealing with vending machines, but real human decisions that matter deeply to organizations and candidates alike.
Is Prediction Just a Fancy Term for Guessing?
Not really. Prediction done properly means using patterns in data to forecast likely outcomes with a level of accuracy that improves over time. In recruiting, it might mean using the profiles of past successful hires to inform which current candidates should be considered more closely. It’s not palm reading or crystal-ball gazing. Instead, it’s closer to having an experienced mentor who has seen thousands of hires and can whisper, “Based on what we’ve learned, this person might really thrive here.”
Predictive analytics lets you stop reacting and start anticipating.
Where Do These Predictions Come From?
Imagine you have a gigantic tapestry woven from threads of past hiring data—applications, interviews, performance reviews, promotions, turnover records, even subtle signals like how quickly a person ramped up in their role.
Predictive analytics sifts through this tapestry.
It looks for recurring shapes and patterns that correlate strongly with success, retention, and team synergy. Machine learning models might notice that candidates with a background in certain projects adapt quicker, or that certain communication styles align better with team culture. This isn’t guesswork; it’s pattern recognition on a massive scale.
- “Data, when carefully interpreted, can whisper truths about the future.” – A lead data scientist once said this while examining decades of hiring logs, and the phrase stuck. It reminds us that the secret sauce is not brute force data crunching alone—it’s how we use insight and discretion to guide the models.
The Quiet Revolution Already Underway
If you talk to a traditional recruiter, they might recount war stories of sifting through piles of resumes by hand, relying on gut feel, or checking off a list of basic qualifications. But as organizations have grown more complex and global, gut instinct alone struggles to keep pace. A whisper in certain HR circles today: “If you’re not using predictive analytics, you’re recruiting in the dark.”
In some places, predictive models have already proven their worth. For example, Machine Hiring has helped companies cut initial screening time by up to 60%. Instead of rummaging through a thousand resumes, a recruiter might focus on the top fifty that the model identifies as promising. It’s not about replacing the human touch; it’s about sharpening it.
From Data Points to Stories
A purely robotic approach would be dull and suspicious. Data is not truth—it’s just a clue. What differentiates a nuanced approach is interpreting these clues like a seasoned detective. A candidate’s educational background might mean one thing in isolation, but combined with their portfolio and past project outcomes, it might tell a deeper story. Predictive analytics can highlight patterns, but recruiters and hiring managers still apply judgment and empathy, considering what the data can’t capture: ambition, personal resilience, or a spark that doesn’t fit neatly into an algorithm.
The Complexity of Culture Fit
A tricky factor: organizational culture. We all talk about it as if it’s obvious, but culture is slippery—more feeling than formula. Yet predictive models are getting better at approximating how a candidate’s communication style, working habits, and values align with a company’s environment. By looking at patterns from top performers—how they communicate, what professional backgrounds they share—models can hint which traits matter. Still, no algorithm should ever be the final arbiter of cultural fit; it can only be a compass pointing roughly north.
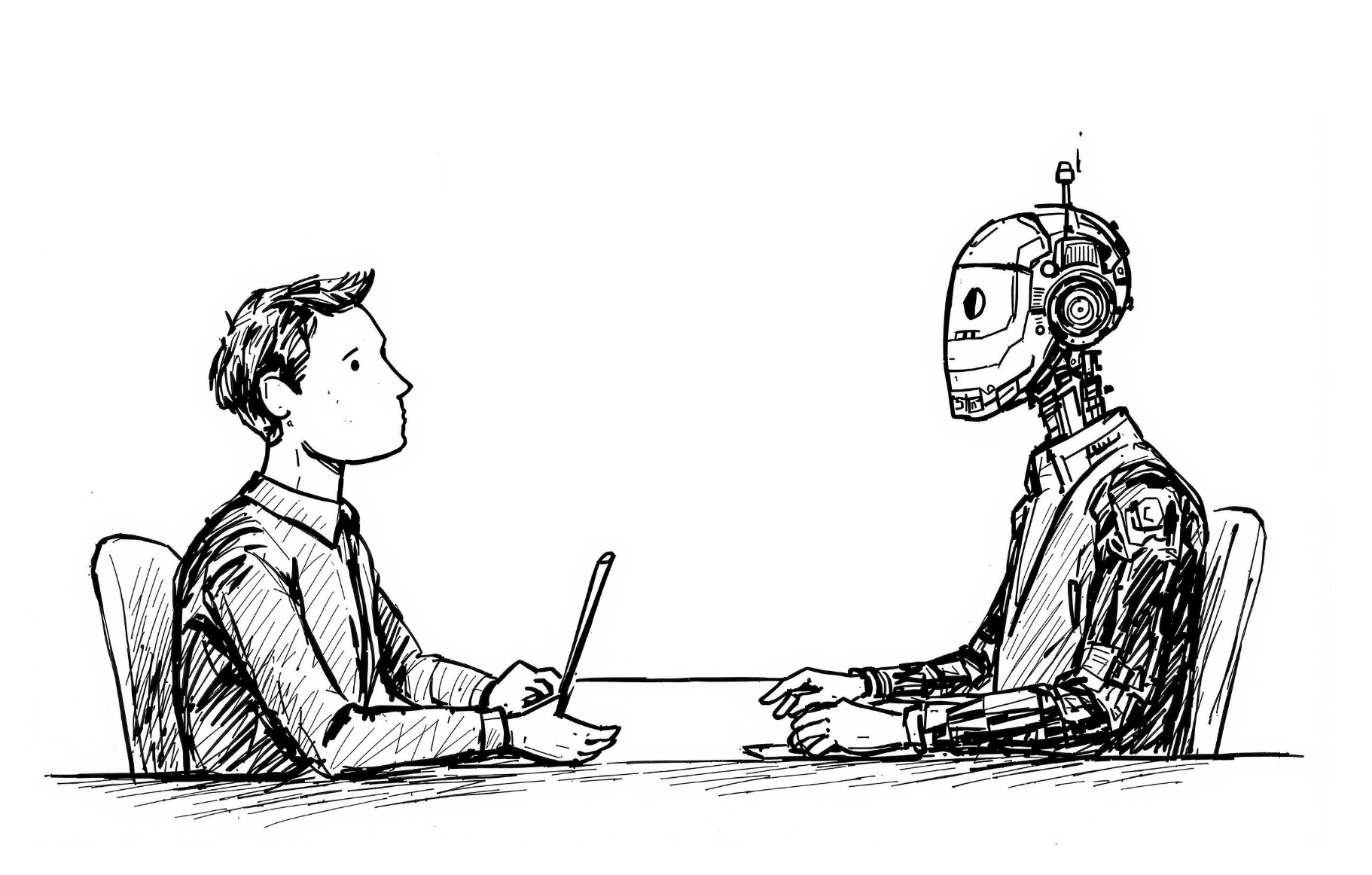
Recruiter: “Okay, we’ve got 300 applicants. Half have the qualifications. Where do I start?”
Predictive Model: “Here. Start with these 20—historically, people with these backgrounds and skill clusters have a 70% better chance of succeeding.”
Recruiter: “So you’re saying I ignore the others?”
Predictive Model: “No, just start here if you’re short on time. I’m suggesting where you may find hidden gems faster.”
The Dark Side of Data: Confronting AI Bias
Let’s pause and turn on a flashlight into a dark corner: bias. If historical hiring data is riddled with unfair preferences, then the predictive model might perpetuate them. A model trained on a past where certain groups were overlooked might erroneously deem those candidates as “not a good fit.” This is a serious issue.
The industry is well aware of this. Many HR and analytics teams now carefully audit their algorithms. Techniques like removing demographic indicators or building fairness constraints into the model are emerging. Some organizations bring in external experts to challenge the models—like shining daylight through stained glass to see if it reflects any hidden patterns that shouldn’t be there.
The Daily Grind—But Faster
We often think of innovation as fireworks. But predictive analytics often hums quietly in the background. It cuts down repetitive tasks: scanning resumes, pre-screening applicants, scheduling interviews. Some systems can even predict which interview questions might differentiate top performers from average candidates based on historical responses.
A moment of honesty: speed matters. Hiring delays can cost time, money, and morale. Shaving days or weeks off the process without losing thoroughness is a big deal. Predictive analytics can triage the talent pool, surfacing strong contenders earlier so the human side of recruitment can do what it does best—connect, evaluate deeply, and make nuanced judgments.
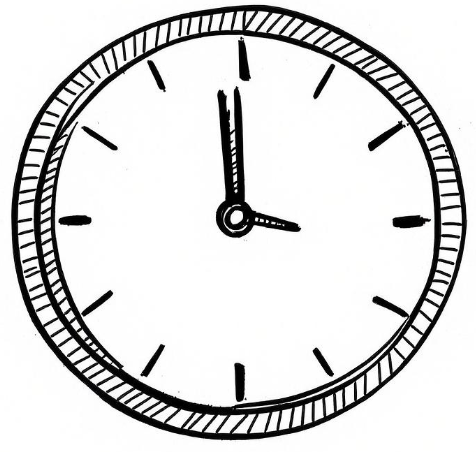
Success Metrics That Actually Matter
The true test is not how fancy the model is, but whether it leads to better outcomes: strong performers who stay longer, contribute more, and fit well with teams. Predictive analytics encourages a long-term lens. Instead of measuring success by how quickly a role was filled, why not consider six months down the road: Is the new hire thriving? Did performance match the model’s forecast?
For instance, Machine Hiring users report that their candidate relevance rating improves by up to 60% when using predictive analytics, leading to better long-term retention rates. Organizations can integrate feedback loops—once the hire is made, track their performance and growth. Feed that data back into the model. Over time, predictive analytics gets smarter. It’s not a static crystal ball; it’s a self-improving compass.
A New Era of Recruitment Refinement
Historically, recruitment relied heavily on gut instincts. Useful instincts, yes, but limited. Predictive analytics acts like reading glasses, sharpening vision. With more clarity, recruiters can see subtle patterns: maybe certain universities produce graduates who excel in creative roles, or maybe people who mention certain portfolio projects adapt faster to challenging environments.
This careful guidance can save organizations from costly mis-hires. Bad hires cost more than money. They affect team morale, slow projects, and can even damage relationships with clients. Mitigating that risk with evidence-based insights is invaluable.
When Data Disagrees with Experience
It can happen: a recruiter is sure a certain profile isn’t ideal, but the model suggests otherwise. This tension can be healthy. Sometimes, challenging human assumptions leads to discovering new talent pools. Other times, the recruiter might know a contextual factor the model can’t see—like a candidate’s unique portfolio that doesn’t fit any known pattern. The best outcomes often arise from dialogue, not deference. Predictive analytics should be a respected advisor, not a dictator.
The Strange Beauty of Human and Machine Collaboration
There’s beauty in blending the algorithm’s structured insight with the human’s creativity and intuition. One sees patterns in data, the other sees patterns in character. For instance, a predictive model might identify that candidates with a certain certification have historically excelled. The recruiter, knowing the field has changed drastically in the last year, might adjust the model’s weighting or seek out new signals. This conversation between analytics and intuition helps both evolve.
As companies scale, hiring volume can surge. Without assistance, it’s easy to drown in resumes and LinkedIn profiles. Predictive analytics can handle a flood of information and present manageable shortlists. In fast-moving industries, a week’s delay might mean losing top talent to competitors. Streamlined pipelines, guided by data, keep the process human yet agile. It’s not about removing phone calls or coffee chats; it’s about ensuring those calls and chats happen with the right people sooner.
Discovering Hidden Talents
Occasionally, predictive analytics can surprise everyone by spotlighting a candidate who, on paper, seems non-traditional. Maybe their background is different, or their career path winding. Traditional filters might’ve passed them over. But the model, recognizing certain subtle characteristics—problem-solving patterns, a knack for learning new tools quickly—might recommend a closer look. This can lead to more diverse hires, bringing fresh perspectives and better team outcomes.
Isn’t This Just Automation?
There’s a misconception that predictive analytics is a fancy way to automate everything and remove humans from the loop. In truth, it’s more like a well-tuned amplifier. The music is human judgment, but the amplifier ensures the notes are clearer, the audience hears the right melodies, and the distortion is minimized. Automation can handle the grunt work: sorting, comparing, filtering. But crucial decisions—like who joins the team—remain deeply human.
Training the Models: The Underrated Work
Behind the scenes, building a predictive model takes effort. Data scientists clean and refine training sets, HR teams label what “success” looks like. It’s not a plug-and-play affair. Models require ongoing maintenance. They must be retrained regularly to reflect changing job demands or new skill requirements. If a company pivots to a new technology stack, yesterday’s success signals may become outdated. Predictive analytics must evolve just like any craft.
The market is swarming with software promising miraculous hiring results: dashboards that highlight candidate potential, tools that claim to predict retention risk, or apps that rank resumes by compatibility. While the brand names differ, the underlying principle is similar—using patterns from historical data to guide future decisions. The challenge is selecting tools that align with your organization’s values, needs, and readiness to handle data responsibly.
While predictive analytics can suggest that person A might outperform person B, it can’t tell you how to respect the individuality of person A or B.
Humans must remember that these candidates are not data points; they are people looking for a place to grow. The moment we forget that, we risk turning recruitment into a grim numbers game. The best organizations see predictive analytics as a helping hand, not a hammer.
A fascinating angle is how historical hiring data can reveal not just what works, but what’s changing. Maybe ten years ago, candidates from a certain field were top performers, but now it’s those who are self-taught and adaptable. Predictive analytics can highlight evolving skill demands and emerging talent pools, warning you that criteria you once prized might no longer predict success. This foresight can guide training, mentorship, and even corporate strategy.
No model is perfect. Sometimes it will recommend a candidate who doesn’t excel, or overlook someone who blossoms into a star. Recognizing uncertainty is key. Savvy recruiters use predictive analytics as one of multiple inputs. Maybe the data suggests a 60% likelihood of a candidate thriving—that leaves room for the recruiter to investigate the other 40%. It’s in that remaining percentage that human intuition often finds unexpected brilliance.
Without predictive analytics, hiring can feel like throwing darts blindfolded. With it, we gain night vision goggles, not x-ray vision. We still see the environment, we just see it more clearly. The ultimate goal: a recruitment process that’s more transparent, fair, and effective. A process where every candidate is considered thoughtfully, and where the organization builds stronger teams faster.
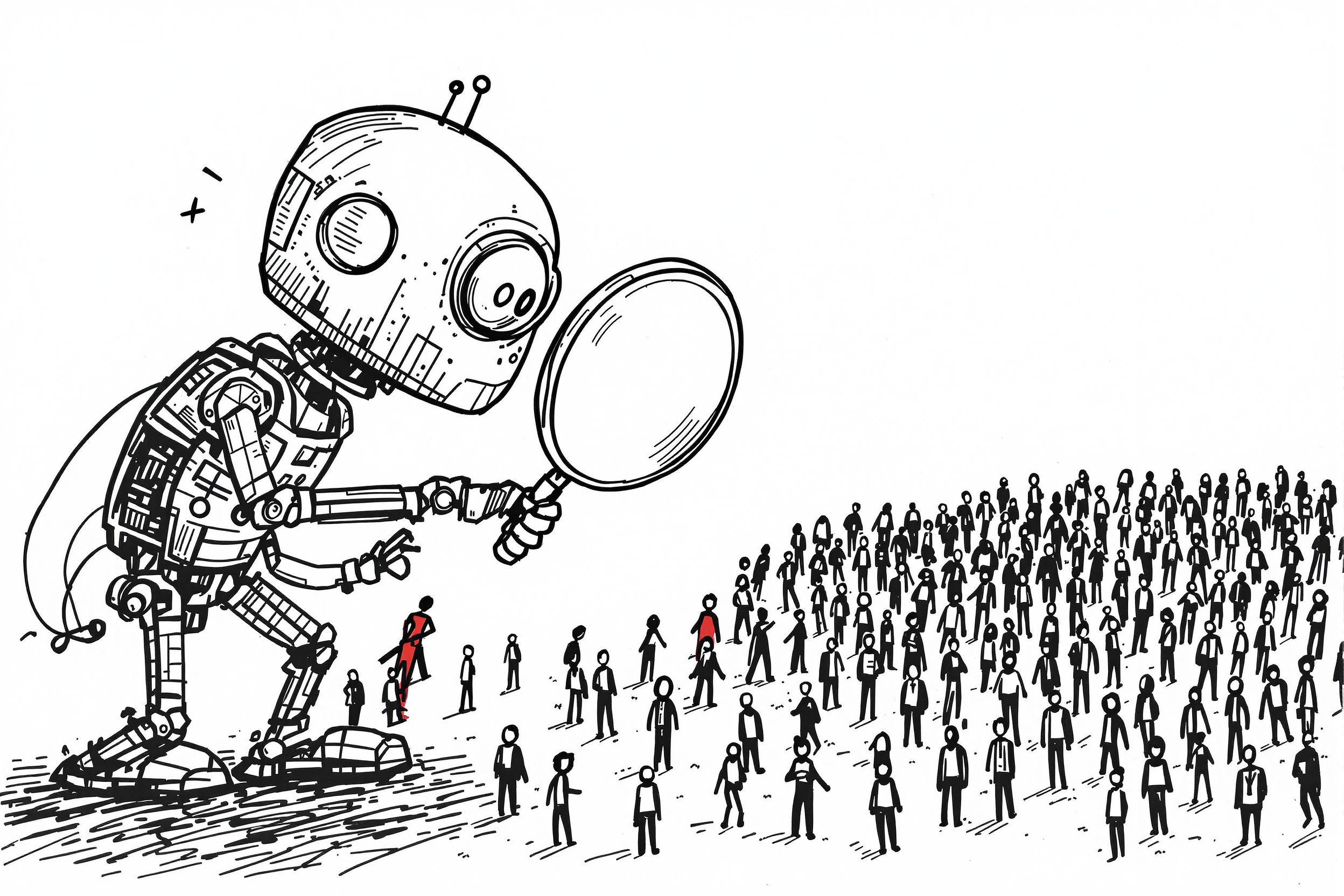
What’s Next?
As technology advances, predictive analytics might incorporate more nuanced data sources—like analyzing communication patterns in emails or team chat tools to see who adapts well to remote work. It might learn to recognize “hidden” skills. It could even help tailor onboarding processes to the strengths and weaknesses of each new hire, ensuring a smoother transition.
There’s talk in some corners of integrating natural language processing to understand candidate motivations in essays or cover letters. Machine Hiring is already pioneering this approach with its AI-powered candidate chat interface and smart interview planning tools.
Others experiment with using predictive models to identify not just who to hire but how to develop them over time. The field is fertile ground for innovation.
Let’s remember the tension between efficiency and empathy. Hiring faster can’t mean ignoring human nuance. Predictive analytics done right expands human capacity. Recruiters get more time to spend on meaningful conversations. Hiring managers reduce guesswork. Candidates feel less like they’re lost in a chaotic lottery. But maintain the balance: treat the models as advisors and never outsource moral responsibility.
Recruiters who’ve integrated predictive analytics often describe a feeling of relief: no more drowning in irrelevant resumes, no more endless cycles of trial-and-error. They enjoy spending more time getting to know candidates who have a genuine shot. Candidates, for their part, might notice a smoother process, quicker responses, and interviews that feel more on-point.
The Laughter at the Vending Machines
Back to that strange dream: the vending machines were an absurd metaphor. Without predictive insights, recruiting can sometimes feel random—tokens spent on uncertain outcomes. But with data-driven models guiding us, we might pick the right “machine” more often, or better yet, learn what’s inside before inserting a coin.
Predictive analytics won’t remove the complexity of human potential, but it can help us navigate it with more grace and insight.
Transform Your Hiring with an AI-Powered Applicant Tracking System
Machine Hiring's ATS helps you leverage the power of predictive analytics to make smarter hiring decisions. Our platform combines advanced AI algorithms with a complete applicant tracking system to help you manage candidates and identify top talent faster than ever before.
Enterprise-Ready ATS Features
A New Chapter Begins
This isn’t the end of the story. Every improvement in predictive analytics introduces new questions: How do we refine the models further? How do we ensure fairness and ethics? Which emerging datasets might give us even clearer signals about future success?
As organizations adopt these tools, they step into a new chapter where the blend of data-driven prediction and human judgment creates a hiring ecosystem more attuned to both the company’s needs and the candidate’s potential. In that delicate dance, we may find something far more human at the heart of our recruiting efforts: understanding, equity, and growth.
Want to learn more about modern recruitment practices? Check out our other articles:
- 5 AI-Powered Recruitment Strategies That Actually Work
- The Art of Selecting Great People
- Mind-Blowing Ways AI is Transforming Recruitment
Transform your recruitment with Machine Hiring - where AI meets expertise. Start your free trial today.